When it comes to the SaaS landscape, especially those in high-revenue SaaS businesses, understanding and predicting customer behavior is more crucial than ever. Luckily, data science provides a window into what a customer might or might not do.
Data science has emerged as a powerful tool, enabling businesses to gain deep insights into their customers' actions and preferences. Leveraging advanced analytics and machine learning algorithms enables companies to anticipate customer needs, enhance user experiences, and drive sustainable growth.
For SaaS businesses earning $1million plus annually, predicting customer behavior isn't just about staying ahead of the competition. It's about creating personalized experiences that foster loyalty and long-term engagement. Data science allows companies to move beyond traditional analytics, providing a more granular view of customer interactions and trends.
Want to learn how data science can be harnessed to predict customer behavior, and reduce churn effectively? Keep reading!
Step 1 - Define Your Objective
The first step in predicting customer behavior using data science is defining clear and actionable objectives. Without a well-defined goal, your efforts can become unfocused, leading to wasted resources and missed opportunities. For SaaS businesses, common objectives include increasing customer retention, identifying upsell opportunities, reducing churn, or enhancing customer satisfaction.
When setting your objectives, it's important to be specific and measurable. For instance, instead of a broad goal like "improve CSAT scores," aim for something specific. For example, "increase customer satisfaction scores by 7% over the next six months."
This clarity will guide your data collection and analysis processes, ensuring you stay on track and achieve meaningful results.
One effective way to define your objectives is to use SMART criteria. SMART stands for:
- Specific
- Measurable
- Achievable
- Relevant
- Time-bound
This framework helps break down your goals into manageable components, making it easier to track progress and make necessary adjustments. Let’s say your goal is to reduce churn. In this case, you might set a specific target like "to reduce churn rate by 15% within the next quarter by identifying at-risk customers and implementing targeted retention strategies."
Align your objectives with your overall business strategy
Your goals should support your long-term vision and drive your company's growth. Involve key stakeholders in the goal-setting process to ensure alignment and buy-in across the organization. This collaboration will help integrate data science initiatives seamlessly into your existing workflows and decision-making processes.
Defining your objectives also involves understanding your customers' needs and pain points. Use customer feedback, surveys, and behavioral data to identify common challenges and areas for improvement. This customer-centric approach ensures that your data science efforts are focused on delivering real value to your users, ultimately driving better engagement and loyalty.
Step 2 - Data Cleansing and Synthesis
Once you've defined your objectives, the next crucial step in predicting customer behavior is data cleansing and synthesis. This process involves refining and integrating data from various sources to ensure accuracy, consistency, and completeness. Clean, high-quality data is the backbone of any successful data science initiative, providing the foundation for reliable analysis and actionable insights.
Data cleansing begins with identifying and rectifying errors and inconsistencies in your datasets. Common issues include duplicate records, missing values, and formatting inconsistencies. Addressing these problems is essential because inaccurate data can lead to misleading conclusions and poor decision-making. Use automated tools and algorithms to streamline the cleansing process, ensuring efficiency and reducing the risk of human error.
Standardize your data
Another important aspect of data cleansing is standardizing your data. This involves converting different data formats into a consistent structure, making it easier to analyze and compare. For example, you might need to standardize date formats, convert currencies, or normalize categorical data. Consistency across your datasets ensures that your analysis is accurate and meaningful.
Data synthesis involves integrating data from multiple sources to create a comprehensive view of your customers. SaaS businesses often collect data from various touchpoints, including CRM systems, web analytics, social media, and customer support platforms. By combining these disparate sources, you can gain a holistic understanding of customer behavior and preferences. Use data integration tools and techniques such as ETL (Extract, Transform, Load) processes to merge and harmonize your data efficiently.
A key challenge in data synthesis is ensuring data compatibility. Different data sources may use varying terminologies, structures, and formats. To address this, establish a unified data schema that standardizes the way data is represented across your organization. This schema acts as a common language, enabling seamless data integration and analysis.
Furthermore, maintaining data quality over time is essential. Implement data governance practices to continuously monitor and improve data quality. Regular audits, validation checks, and feedback loops help ensure that your data remains accurate and up-to-date, supporting ongoing data science initiatives.
No matter how you look at it, data cleansing and synthesis are critical steps in preparing your data for predictive analysis. When your data is accurate, consistent, and integrated, you can build a solid foundation for predicting customer behavior and driving informed business decisions.
Step 3 - Building a Customer Profile
After cleansing and synthesizing your data, the next step in predicting customer behavior is building comprehensive customer profiles. These profiles provide detailed insights into your customers' demographics, behaviors, preferences, and pain points, enabling more accurate predictions and personalized interactions.
To build effective customer profiles, start by gathering relevant data from various sources. This includes demographic information such as age, gender, and location, as well as behavioral data like purchase history, website interactions, and customer support interactions. Combining these data points helps create a holistic view of each customer, allowing for a more nuanced analysis.
Customer segmentation helps you organize and visualize data
Segmenting your customers into distinct groups based on shared characteristics or behaviors enables you to tailor your marketing and engagement strategies to each segment's unique needs. For example, you might create segments based on purchase frequency, product preferences, or engagement levels. This targeted approach ensures that your efforts are more relevant and effective, driving better results.
To enhance the accuracy and depth of your customer profiles, leverage advanced analytics and machine learning techniques. These technologies can identify patterns and correlations in your data that might not be apparent through manual analysis. For instance, clustering algorithms can group customers based on complex behavioral patterns, while predictive models can forecast future actions based on past behavior. By incorporating these insights into customer profiles, you can make more informed decisions and anticipate customer needs more effectively.
Consider incorporating qualitative data into your customer profiles
Customer feedback, surveys, and reviews provide valuable context that complements quantitative data. This qualitative information helps you understand the underlying motivations and emotions driving customer behavior, enriching customer profiles and enabling more empathetic engagement.
Maintaining up-to-date customer profiles is crucial for ongoing success. Customer behavior and preferences can change over time, so it's important to regularly update your customer profiles with fresh data. Implementing automated data collection and analysis processes ensures that your customer profiles remain current, providing a reliable foundation for your predictive efforts.
Finally, ensure that your customer profiles are easily accessible and usable by your team. Invest in user-friendly tools and platforms that allow team members to view, analyze, and act on profile data. This accessibility fosters a data-driven culture within your organization, empowering your team to leverage insights effectively in their daily operations.
Step 4 - Predict Behavior Through Segmentation: Anticipating Churn
Predicting customer behavior effectively requires a strategic approach to segmentation. Tailor your marketing and engagement strategies to meet the specific needs of each group. Divide your customer base into distinct segments based on shared characteristics. This targeted approach not only enhances customer satisfaction but also drives better business outcomes.
The first step in segmentation is identifying the key attributes that differentiate your customers. These attributes can include demographic factors such as age, gender, and location, as well as behavioral data like purchase history, product usage, and engagement levels. Advanced analytics tools and machine learning algorithms can help you uncover patterns and correlations within your data, guiding your segmentation efforts.
One effective segmentation strategy is RFM (Recency, Frequency, Monetary) analysis. This method categorizes customers based on how recently they made a purchase, how often they buy, and how much they spend. RFM analysis helps you identify high-value customers, loyal repeat buyers, and those at risk of churning. By understanding these segments, you can develop tailored strategies to nurture each group, such as personalized offers for high-value customers or re-engagement campaigns for those who haven't purchased recently.
Another powerful approach is behavioral segmentation, which focuses on customers' actions and interactions with your products or services. This can include website visits, email opens, and social media engagement. By analyzing these behaviors, you can create segments that reflect different stages of the customer journey, from awareness to consideration to purchase. This allows you to deliver relevant content and offers at the right time, improving conversion rates and customer satisfaction.
Predictive analytics also plays a crucial role in segmentation. By applying machine learning models to your customer data, you can forecast future behaviors and trends. For example, predictive models can help identify which customers are most likely to churn, allowing you to take proactive measures to retain them. Similarly, you can predict which customers are most likely to respond to specific marketing campaigns, enabling you to allocate resources more effectively.
Anticipating churn is a critical aspect of segmentation. High churn rates can significantly impact your business's revenue and growth. By segmenting customers based on their likelihood to churn, you can implement targeted retention strategies. For instance, you might offer exclusive discounts or personalized support to at-risk customers, increasing their loyalty and reducing churn.
Effective segmentation requires continuous monitoring and refinement. Customer behavior and preferences can change over time, so it's important to regularly update your segments with fresh data. Implementing automated tools for data collection and analysis ensures that your segmentation remains accurate and relevant, providing a solid foundation for your predictive efforts.
FAQs - Predicting Customer Behavior for Saas Growth
With a clear understanding of the predictive analytics framework, you may be wondering about practical implementation for your SaaS business. Let's address the most frequently asked questions about leveraging data science for customer behavior prediction and churn reduction.
How exactly can data science predict SaaS customer behavior?
Data science combines historical customer data, machine learning algorithms, and behavioral analytics to identify patterns that indicate future actions. This helps you anticipate customer needs, predict potential churn risks, and identify opportunities for expansion—all before they happen.
What specific business problems can predictive analytics solve?
The primary benefits include churn reduction, increasing customer lifetime value, identifying upsell opportunities, and optimizing customer engagement strategies. Predicting SaaS customer behavior patterns gives you the ability to proactively address issues before they impact your revenue.
What steps should I take to start implementing predictive analytics for SaaS customer success?
Begin by:
- Collecting and cleaning your customer data
- Identifying key metrics and behaviors to track
- Implementing basic analytics tools
- Developing prediction models for specific outcomes
- Creating response strategies for different scenarios
How does predicting customer behavior for SaaS growth work (i.e. how does it improve my bottom line)?
Understanding and anticipating customer behavior directly affects your revenue stability and growth potential. When you can predict which customers might leave or need attention, you can take proactive steps to retain them, thereby protecting and growing your recurring revenue.
Is implementing predictive analytics complicated?
While the underlying technology can be complex, working with data science experts can make implementation straightforward. The key is starting with clear objectives and gradually building your predictive capabilities based on your specific business needs.
What kind of ROI can I expect from investing in predictive analytics?
Companies implementing predictive analytics typically see improvements in key metrics like:
- Reduced churn rates
- Increased customer lifetime value
- Higher conversion rates
- More efficient resource allocation
- Better customer satisfaction scores
Partnering with Data Science Experts
Predicting customer behavior through data science is a game-changer for SaaS businesses, enabling them to understand and anticipate their customers' needs with precision. If you’re running a high-revenue SaaS business, the keys to success lie in defining clear objectives, cleansing and synthesizing data, building comprehensive customer profiles, and implementing effective segmentation. With data science experts on your side, you can drive meaningful engagement and sustainable growth.
Working with experts like us at Inturact, and our partner InnerTrends can further enhance your data-driven initiatives. We provide the tools, insights, and support necessary to leverage data science effectively, ensuring you stay ahead in the competitive SaaS landscape. With our help, you can make informed decisions, improve customer satisfaction, and achieve long-term success.
Ready to unlock the full potential of data science for your SaaS business? Join forces with Inturact and InnerTrends to transform your customer engagement strategy and drive unparalleled growth. Start your journey today and see the difference data science can make.
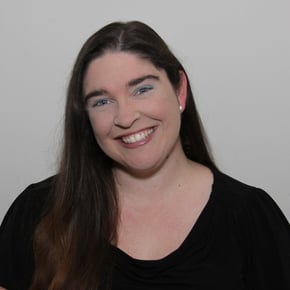